Rheumatic, autoimmune, and autoinflammatory diseases are complex processes regulated at multiple biological levels. The emerging field of omics allows researchers to gather insights across the biological spectrum that have the potential to revolutionize the collective understanding of disease states and the management of patients.
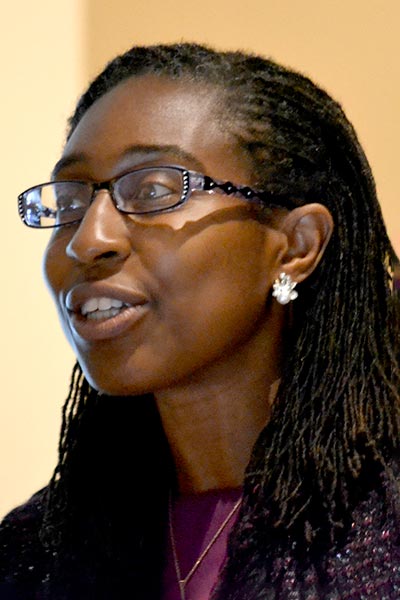
At ACR Convergence 2022, Onyinye Iweala, MD, PhD, Assistant Professor of Medicine, University of North Carolina at Chapel Hill (UNC) Thurston Arthritis Research Center (TARC), and Director, Med/Allergy Mast Cell Disorders Program, and UNC TARC biostatistician Liubov Arbeeva, MSc, delivered an overview of different omics data, visualization techniques, and resources for analysis of high-dimensional data in Stats Bootcamp III. The Monday, November 14, session is available for on-demand viewing for registered ACR Convergence participants through October 31, 2023, on the virtual meeting website.
Using omics data in rheumatology and immunology research enables professionals to understand biological systems or disease states, link omics-based measurements with clinical outcomes of interest, and glean mechanistic information from complex biological networks. This leads to a greater understanding of inter- and intra-individual pathologic diversity.
“All of this (omics data analysis) is toward the goal of designing predictive or prognostic models that we can use to improve diagnosis and treatment for our patients,” Dr. Iweala explained.
The omics approach selected often depends on tissue type. With knee tissues or cartilage, many studies focus on genomics or transcriptomics. Conversely, when biological fluids such as plasma or serum are studied, the research analyzes proteomics or metabolomics.
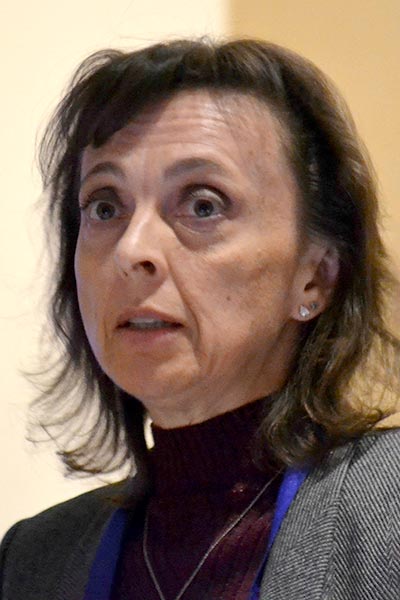
Dr. Iweala visualized several omics data approaches, including bulk transcriptomics profiling and techniques focused on characterizing individual cells. She explained that handling vast quantities of multidimensional data requires specialized statistical and data visualization techniques. There is also the challenge of linking the genotype and phenotype, which researchers overcome by leveraging integrative omics approaches.
“When you start integrating data from these different omics approaches, instead of pieces of the puzzle, you start to get a sense of the whole picture,” Dr. Iweala said.
An almost unlimited number of statistical models can be used to analyze this data. When dealing with large-scale, high-dimensional data at this level, dimension reduction makes analysis less time-consuming.
“We can find lower-dimensional subspace into which the majority of our data is mapped, and it is very helpful if you need to identify outliers or technical sources of variation,” Ms. Arbeeva further explained.
To illustrate, she explored the advantages and limitations of various visualization methods, such as scatter plots, profile plots, and heat maps.
She then expounded on how to classify omics analyses. All models can be classified as unsupervised or supervised, depending on whether an outcome is present. The statistical models can also be classified depending on the unit. Researchers can select an individual gene, gene set, or gene-network-based approach in these cases. Finally, marginal analyses allow researchers to study one unit at a time, while joint analyses are performed when a large number of units are collapsed.
Dr. Iweala and Ms. Arbeeva emphasized the importance of robust study design to maximize the precision of measurements and optimize associated research costs. The involvement of trained biostatisticians, bioinformaticians, or genetic epidemiologists is vital at all stages of omics data research.
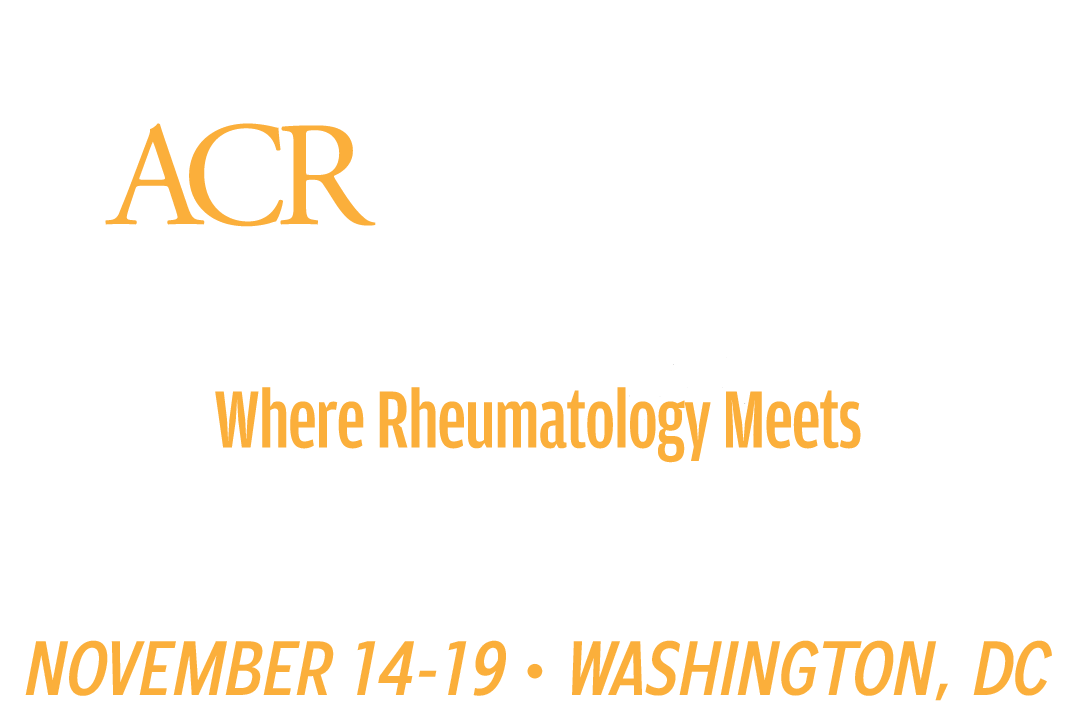
Registered ACR Convergence 2024 Participants:
Watch the Replay
Select ACR Convergence 2024 scientific sessions are available to registered participants for on-demand viewing through October 10, 2025. Log in to the meeting website to continue your ACR Convergence experience.