The performance and accuracy of screening and diagnostic tests are key workflow considerations that should be evaluated regularly. In Stats Bootcamp I, Carolina Alvarez, MS, Biostatistician at the University of North Carolina at Chapel Hill, and Jinoos Yazdany, MD, MPH, Professor of Medicine and Division Chief at the University of California, San Francisco, General Hospital, discussed the most effective methods to analyze the efficiency of various clinical testing strategies. The session is available for on-demand viewing for registered ACR Convergence participants through October 31, 2023, on the virtual meeting website.
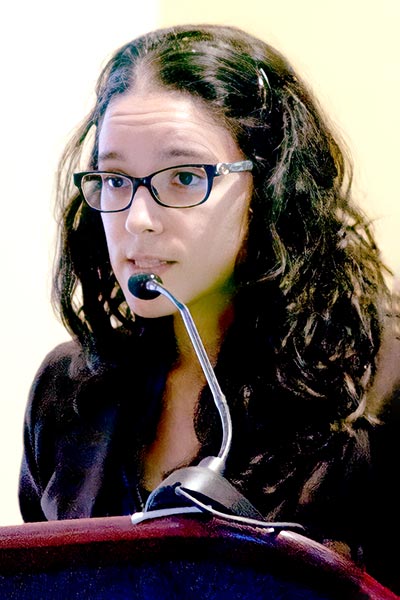
Reference standard tests are considered the current best available analytical tools for clinicians, but they can be expensive, invasive, or require long wait times for results. While alternative index tests might be conclusively faster or cheaper, professionals must perform a diagnostic accuracy study to evaluate performance concerning a specific target condition.
Alvarez reviewed how to calculate key measurements in binary and dichotomous testing comparisons. Sensitivity, or the true positive rate of a test, measures how well the index test detects the features of a given disease. Conversely, specificity measures how well the comparison test rules out these features.
“Positive and negative likelihood ratios inform on the certainty of diagnosis,” Alvarez explained.
While these properties are important values for clinicians, they fail to account for the prevalence of disease in a defined population. When prevalence must be factored in, the positive and negative predictive values should be calculated and plotted to verify the accuracy of the diagnosis, Alvarez said.
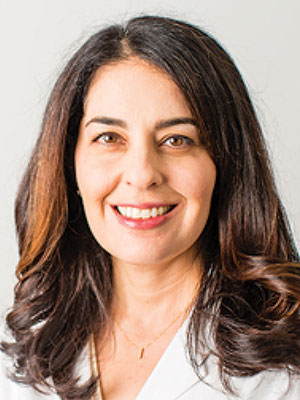
Professionals must also consider whether their units of analysis are correlated or independent. For example, if a researcher analyzed joints in the human foot, two feet from the same person would be categorized as correlated data. Generalized estimating equations can be utilized to account for correlating data when producing estimates and their standard errors, Alvarez noted.
She also analyzed the factors that drive sample size and power considerations in testing.
“If your outcome is of low prevalence, sensitivity is typically going to be expected to drive your sample size estimate,” Alvarez said. “If you had higher prevalence, it would be specificity.”
The quality of reporting for diagnostic accuracy tests can often contain significant gaps. Sources of bias can include inappropriate gold standards, unblinded reviews of test results, and spectrum bias. To improve the quality of reporting of diagnostic accuracy studies, Dr. Yazdany advocated for the use of the Standards for Reporting Diagnostic Accuracy (STARD) checklist.
“As an author, you can use this checklist to make sure that you have complete and transparent reporting of your study,” Dr. Yazdany said. “As a peer reviewer or editor, you can use the checklist to actually judge the information in a manuscript.”
The 30-item checklist follows the format of a manuscript and can be an effective tool for measuring the quality of a report before one decides to implement its suggested clinical practices, she said. The checklist forces the author or reviewer to explicitly specify factors such as study design, limitations, and testing methods that can unintentionally affect research study results.
“Using the STARD checklist consistently will help ensure that our studies have a greater impact,” Dr. Yazdany concluded.
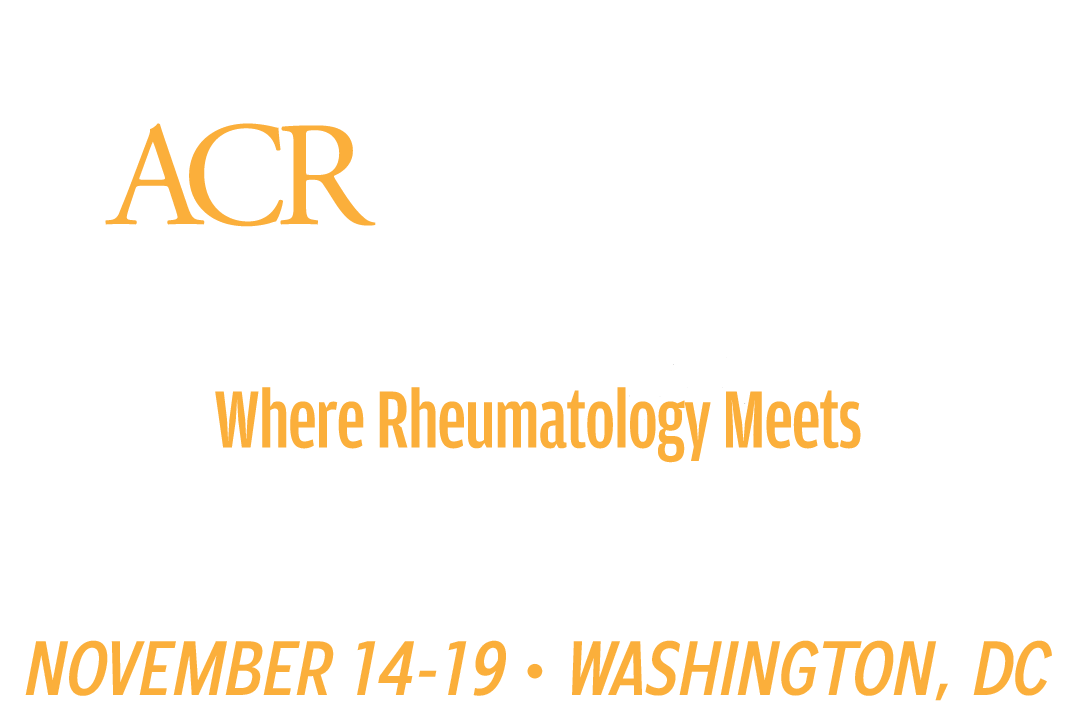
Registered ACR Convergence 2024 Participants:
Watch the Replay
Select ACR Convergence 2024 scientific sessions are available to registered participants for on-demand viewing through October 10, 2025. Log in to the meeting website to continue your ACR Convergence experience.